DT-ITS is one of the Most Attractive Workplaces!
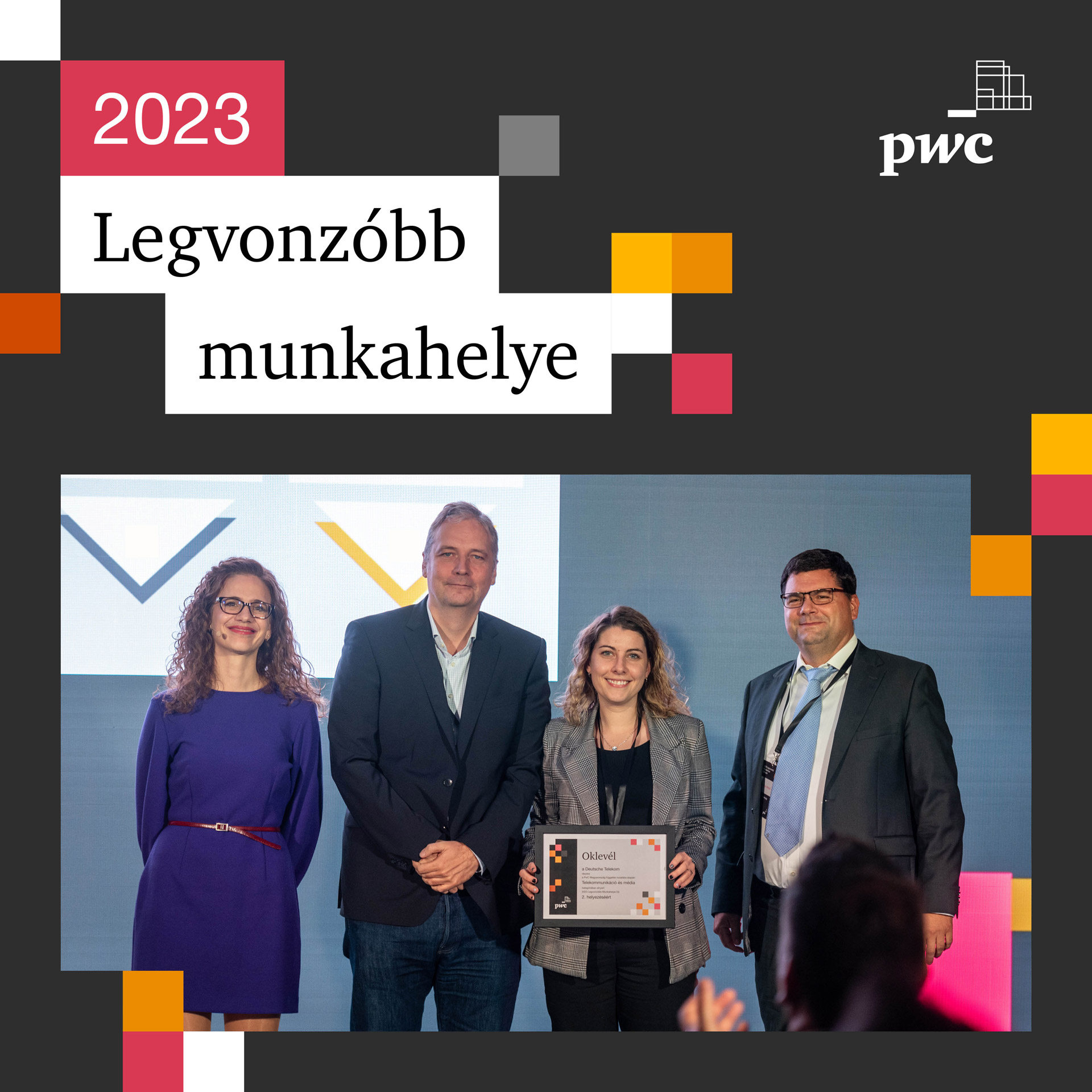
DT-ITS has become the second most attractive workplace in the Telecommunications and Media category in PwC’s 2023 Labour Market Survey.
This year, PwC Hungary used an online questionnaire to explore the job preferences of more than 80,000 Hungarian respondents – young people and experienced professionals.
According to the survey, human aspects have become more important and employees are more focused on maintaining work-life balance. Moreover, the growing importance of job content, team and leadership is also evident from the responses, while increasing the base salary rather than job stability has become a preference.
In addition to exploring workplace preferences, the Most Attractive Workplace of the Year awards were presented in a total of ten industry categories and one overall category based on the ratings of survey respondents, with DT-ITS coming second in the telecoms category.
source: https://www.pwc.com/hu/hu/sajtoszoba/2023/munkaeropiaci_preferencia_felmeres_2023.html
További hírek
The Copernicus Project: Revolutionizing Climate Data Collection and Analysis
In a special edition of the Deutsche Telekom IT Solutions Unmute All podcast, the spotlight was on the Copernicus project, an ambitious and transformative initiative that promises to revolutionize climate research and data collection. Hosted by Péter Civin, the...
End of the school year in Pécs and Szendrőlád
We have closed the academic year in our educational programmes which are part of our CSR strategy. The students in Pécs and Szendrőlád celebrated the end of the school year with a little show and thanked their volunteer teachers for their work. This was the seventh...
Here are five answers to why feeding call center tickets to AI is beneficial!
Hungarian developers optimized Deutsche Telekom's language model. Integrating artificial intelligence (AI) into customer service can bring radical changes, especially in call centers. Voice-based AI technologies automate processes and enhance customer interactions....